News Story
New methodology to estimate neural activity published by eLife
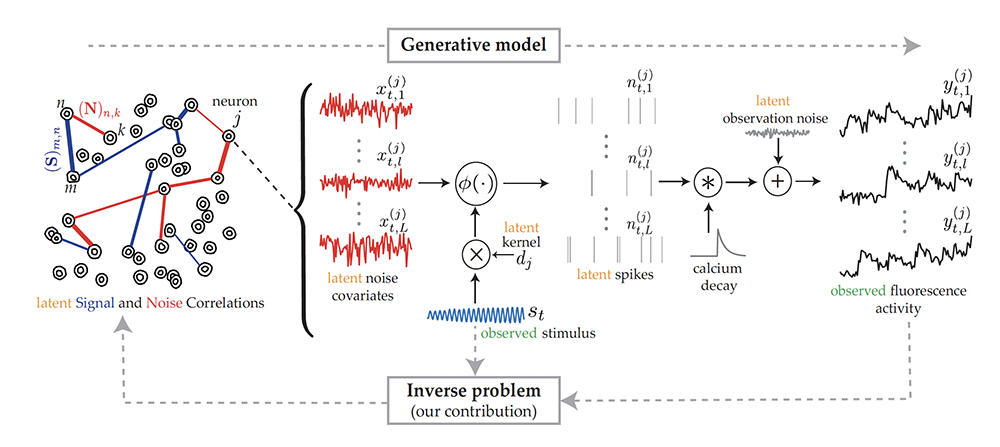
Figure 1 from the paper (click to enlarge). The proposed generative model and inverse problem. Observed (green) and latent (orange) variables pertinent to the jth neuron are indicated, according to the proposed model for estimating the signal (blue) and noise (red) correlations from two-photon calcium fluorescence observations.
Direct extraction of signal and noise correlations from two-photon calcium imaging of ensemble neuronal activity is a new neuroscience article written by six students and faculty associated with ISR. The paper proposes a methodology to explicitly model and directly estimate signal and noise correlations of the brain’s neural activity from two-photon fluorescence observations, without requiring intermediate spike deconvolution.
The paper has been published as a tools and resources article on the open science and open technology innovation website of the non-profit scientific research organization eLife. Tools and resources articles allow authors to publish the details of new experimental techniques, datasets, software tools, and other resources.
Lead author Anuththara Rupasinghe is a Ph.D. student of Associate Professor Behtash Babadi (ECE/ISR), another of the paper’s authors. Former ISR Postdoctoral Researcher Nikolas Francis is another of the authors. He is now a University of Maryland assistant professor with a joint appointment in Biology and the Brain and Behavior Institute. Rounding out the writing team is alum Zac Bowen (2021 Ph.D. Biophysics), a former student of Patrick Kanold (formerly Biology/ISR and currently on the faculty of Johns Hopkins University). Kanold is also an author on the paper, and his current advisee, UMD Biology Ph.D. student Ji Liu, rounds out the list of contributors.
Neuronal activity correlations are key to understanding how populations of neurons collectively encode information. These correlations provide insights into the functional architecture and computations carried out by neuronal networks. Neuronal activity correlations are often categorized in two groups: signal correlations and noise correlations. Given two neurons, signal correlation quantifies the similarity of neural responses that are time-locked to a repeated stimulus across trials, whereas noise correlation quantifies the stimulus-independent trial-to-trial variability shared by neural responses that are believed to arise from common latent inputs.
Two-photon calcium imaging has become increasingly popular in recent years to record in vivo neural activity simultaneously from hundreds of neurons. This technology takes advantage of intracellular calcium flux mostly arising from spiking activity and captures calcium signaling in neurons in living animals using fluorescence microscopy. The observed fluorescence traces of calcium concentrations, however, are indirectly related to neuronal spiking activity. Extracting spiking activity from fluorescence traces is a challenging signal deconvolution problem and has been the focus of active research.
Existing methods for inferring correlations from these data face several challenges. First, the observations of spiking activity produced by two-photon imaging are temporally blurred and noisy. Second, even if the spiking data were perfectly recovered via deconvolution, inferring network-level features from binary spiking data is a challenging task due to the non-linear relation of neuronal spiking to endogenous and exogenous inputs.
The authors propose a methodology to directly estimate both signal and noise correlations from two-photon imaging observations, without requiring an intermediate step of spike deconvolution. They pose the problem under the commonly used experimental paradigm in which neuronal activity is recorded during trials of a repeated stimulus. We avoid the need to perform spike deconvolution by integrating techniques from point processes and state-space modeling that explicitly relate the signal and noise correlations to the observed fluorescence traces in a multi-tier model. This casts signal and noise correlations within a parameter estimation setting. To solve the resulting estimation problem in an efficient fashion, the authors develop a solution method based on variational inference, by combining techniques from Pólya-Gamma augmentation and compressible state-space estimation. They also provide theoretical guarantees on the bias and variance performance of the resulting estimator.
The proposed method can be used as a robust and scalable alternative to existing approaches for extracting signal and noise correlations from two-photon imaging data. It also can be used as groundwork for incorporating other notions of correlation such as the connected correlation function, and to account for non-Gaussian and higher order structures arising from spatiotemporal interactions. Other possible extensions include leveraging variational inference beyond the mean-field regime, extension to time-varying correlations that underlie rapid task-dependent dynamics, and extension to non-linear models such as those parameterized by neural networks.
Published November 18, 2021